Latin square
Displaying a

Latin square, this
stained glass window honors
Ronald Fisher, whose
Design of Experiments discussed Latin squares. Fisher's student, A. W. F. Edwards, designed this window for Caius College, Cambridge.
In the combinatorics and statistics, a Latin square is an n × n table filled with n different symbols in such a way that each symbol occurs exactly once in each row and exactly once in each column. Here is an example:
In the design of experiments, Latin squares are a special case of row-column designs for two blocking factors[1]: Many row-column designs are constructed by concatenating Latin squares.[2] In algebra, Latin squares are generalizations of groups; in fact, Latin squares are characterized as being the multiplication tables (Cayley tables) of quasigroups. Other applications include error correcting codes.
The name Latin square originates from Leonhard Euler, who used Latin characters as symbols.
A Latin square is said to be reduced (also, normalized or in standard form) if its first row and first column are in natural order. For example, the Latin square above is reduced because both its first row and its first column are 1,2,3 (rather than 3,1,2 or any other order). We can make any Latin square reduced by permuting (reordering) the rows and columns.
Properties
Orthogonal array representation
If each entry of an n × n Latin square is written as a triple (r,c,s), where r is the row, c is the column, and s is the symbol, we obtain a set of n2 triples called the orthogonal array representation of the square. For example, the orthogonal array representation of the first Latin square displayed above is
- { (1,1,1),(1,2,2),(1,3,3),(2,1,2),(2,2,3),(2,3,1),(3,1,3),(3,2,1),(3,3,2) },
where for example the triple (2,3,1) means that in row 2 and column 3 there is the symbol 1. The definition of a Latin square can be written in terms of orthogonal arrays:
- A Latin square is the set of all triples (r,c,s), where 1 ≤ r, c, s ≤ n, such that all ordered pairs (r,c) are distinct, all ordered pairs (r,s) are distinct, and all ordered pairs (c,s) are distinct.
For any Latin square, there are n2 triples since choosing any two uniquely determines the third. (Otherwise, an ordered pair would appear more than once in the Latin square.)
The orthogonal array representation shows that rows, columns and symbols play rather similar roles, as will be made clear below.
Equivalence classes of Latin squares
Many operations on a Latin square produce another Latin square (for example, turning it upside down).
If we permute the rows, permute the columns, and permute the names of the symbols of a Latin square, we obtain a new Latin square said to be isotopic to the first. Isotopism is an equivalence relation, so the set of all Latin squares is divided into subsets, called isotopy classes, such that two squares in the same class are isotopic and two squares in different classes are not isotopic.
Another type of operation is easiest to explain using the orthogonal array representation of the Latin square. If we systematically and consistently reorder the three items in each triple, another orthogonal array (and, thus, another Latin square) is obtained. For example, we can replace each triple (r,c,s) by (c,r,s) which corresponds to transposing the square (reflecting about its main diagonal), or we could replace each triple (r,c,s) by (c,s,r), which is a more complicated operation. Altogether there are 6 possibilities including "do nothing", giving us 6 Latin squares called the conjugates (also parastrophes) of the original square.
Finally, we can combine these two equivalence operations: two Latin squares are said to be paratopic, also main class isotopic, if one of them is isotopic to a conjugate of the other. This is again an equivalence relation, with the equivalence classes called main classes, species, or paratopy classes. Each main class contains up to 6 isotopy classes.
Number
There is no known easily-computable formula for the number L(n) of n × n Latin squares with symbols 1,2,...,n. The most accurate upper and lower bounds known for large n are far apart. One classic result is
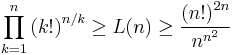
(this given by van Lint and Wilson).
Here we will give all the known exact values. It can be seen that the numbers grow exceedingly quickly. For each n, the number of Latin squares altogether (sequence A002860 in OEIS) is n! (n-1)! times the number of reduced Latin squares (sequence A000315 in OEIS).
The numbers of Latin squares of various sizes
n |
reduced Latin squares of size n |
all Latin squares of size n |
1 |
1 |
1 |
2 |
1 |
2 |
3 |
1 |
12 |
4 |
4 |
576 |
5 |
56 |
161280 |
6 |
9408 |
812851200 |
7 |
16942080 |
61479419904000 |
8 |
535281401856 |
108776032459082956800 |
9 |
377597570964258816 |
5524751496156892842531225600 |
10 |
7580721483160132811489280 |
9982437658213039871725064756920320000 |
11 |
5363937773277371298119673540771840 |
776966836171770144107444346734230682311065600000 |
For each n, each isotopy class (sequence A040082 in OEIS) contains up to (n!)3 Latin squares (the exact number varies), while each main class (sequence A003090 in OEIS) contains either 1, 2, 3 or 6 isotopy classes.
Equivalence classes of Latin squares
n |
main classes |
isotopy classes |
1 |
1 |
1 |
2 |
1 |
1 |
3 |
1 |
1 |
4 |
2 |
2 |
5 |
2 |
2 |
6 |
12 |
22 |
7 |
147 |
564 |
8 |
283657 |
1676267 |
9 |
19270853541 |
115618721533 |
10 |
34817397894749939 |
208904371354363006 |
Examples
We give one example of a Latin square from each main class up to order 5.
They present, respectively, the multiplication tables of the following groups:
- {0} - the trivial 1-element group
- the binary group
- cyclic group of order 3
- the Klein four-group
- cyclic group of order 4
- cyclic group of order 5
- the last one is an example of a quasigroup, or rather a loop, which is not associative.
Applications
Error correcting codes
Sets of Latin squares that are orthogonal to each other have found an application as error correcting codes in situations where communication is disturbed by more types of noise than simple white noise, such as when attempting to transmit broadband Internet over powerlines.[3][4][5]
Firstly, the message is sent by using several frequencies, or channels, a common method that makes the signal less vulnerable to noise at any one specific frequency. A letter in the message to be sent is encoded by sending a series of signals at different frequencies at successive time intervals. In the example below, the letters A to L are encoded by sending signals at four different frequencies, in four time slots. The letter C for instance, is encoded by first sending at frequency 3, then 4, 1 and 2.
The encoding of the twelve letters are formed from three Latin squares that are orthogonal to each other. Now imagine that there's added noise in channels 1 and 2 during the whole transmission. The letter A would then be picked up as:

In other words, in the first slot we receive signals from both frequency 1 and frequency 2; while the third slot has signals from frequencies 1, 2 and 3. Because of the noise, we can no longer tell if the first two slots were 1,1 or 1,2 or 2,1 or 2,2. But the 1,2 case is the only one that yields a sequence matching a letter in the above table, the letter A. Similarly, we may imagine a burst of static over all frequencies in the third slot:

Again, we are able to infer from the table of encodings that it must have been the letter A being transmitted. The number of errors this code can spot is one less than the number of time slots. It has also been proved that if the number of frequencies is a prime or a power of a prime, the orthogonal Latin squares produce error detecting codes that are as efficient as possible.
Mathematical puzzles
The problem of determining if a partially filled square can be completed to form a Latin square is NP-complete.[6]
The popular Sudoku puzzles are a special case of Latin squares; any solution to a Sudoku puzzle is a Latin square. Sudoku imposes the additional restriction that nine particular 3×3 adjacent subsquares must also contain the digits 1–9 (in the standard version). The more recent KenKen puzzles are also examples of latin squares.
Heraldry
The Latin square also figures in the blazon of the arms of the Statistical Society of Canada.[7] Also, it appears in the logo of the International Biometric Society.[8]
See also
- Latin hypercube sampling
- Graeco-Latin square
- Magic Square
- Problems in Latin squares
- Small Latin squares and quasigroups
- Mathematics of Sudoku
- Futoshiki
- Rook's graph, a graph that has Latin squares as its colorings.
- Eight queens puzzle
- Block design
- Word square
Notes
- ↑
- ↑
- Raghavarao, Damaraju (1988). Constructions and Combinatorial Problems in Design of Experiments (corrected reprint of the 1971 Wiley ed.). New York: Dover.
- Raghavarao, Damaraju and Padgett, L.V. (2005). Block Designs: Analysis, Combinatorics and Applications. World Scientific.
- Shah, Kirti R.; Sinha, Bikas K. (1989). "4 Row-Column Designs". Theory of Optimal Designs. Lecture Notes in Statistics. 54. Springer-Verlag. pp. 66–84. MR1016151. ISBN 0-387-96991-8.
- Shah, K. R.; Sinha, Bikas K. (1996). "Row-column designs". In S. Ghosh and C. R. Rao. Design and analysis of experiments. Handbook of Statistics. 13. Amsterdam: North-Holland Publishing Co.. pp. 903–937. MR1492586. ISBN 0-444-82061-2.
- Street, Anne Penfold and Street, Deborah J. (1987). Combinatorics of Experimental Design. Oxford U. P. [Clarendon]. pp. 400+xiv. ISBN 0198532563.
- ↑ C.J. Colbourn, T. Kløve, and A.C.H. Ling, Permutation arrays for powerline communication, IEEE Trans. Inform. Theory, vol. 50, pp. 1289-1291, 2004.
- ↑ Euler's revolution, New Scientist, 24th of March 2007, pp 48-51
- ↑ Sophie Huczynska, Powerline communication and the 36 officers problem, Philosophical Transactions of the Royal Society A, vol 364, p 3199.
- ↑ C. Colbourn (1984). "The complexity of completing partial latin squares". Discrete Applied Mathematics 8: 25–30. doi:10.1016/0166-218X(84)90075-1.
- ↑ [1]
- ↑ [2]
References
- Bailey, R.A. (2008). "6 Row-Column designs and 9 More about Latin squares". Design of Comparative Experiments. Cambridge University Press. MR2422352. ISBN 978-0-521-68357-9. http://www.maths.qmul.ac.uk/~rab/DOEbook. Pre-publication chapters are available on-line.
- Dénes, J.; Keedwell, A. D. (1974). Latin squares and their applications. New York-London: Academic Press. pp. 547. MR351850.
- Dénes, J. H.; Keedwell, A. D. (1991). Latin squares: New developments in the theory and applications. Annals of Discrete Mathematics. 46. Amsterdam: Academic Press. pp. xiv+454. MR1096296. ISBN 0444888993.
- Hinkelmann, Klaus; Kempthorne, Oscar (2008). Design and Analysis of Experiments. I , II (Second ed.). Wiley. MR2363107. ISBN 978-0-470-38551-7. http://eu.wiley.com/WileyCDA/WileyTitle/productCd-0470385510.html.
- Laywine, Charles F.; Mullen, Gary L. (1998). Discrete mathematics using Latin squares. Wiley-Interscience Series in Discrete Mathematics and Optimization. New York: John Wiley & Sons, Inc.. pp. xviii+305. MR1644242. ISBN 0-471-24064-8.
- Shah, Kirti R.; Sinha, Bikas K. (1989). "4 Row-Column Designs". Theory of Optimal Designs. Lecture Notes in Statistics. 54. Springer-Verlag. pp. 66–84. MR1016151. ISBN 0-387-96991-8.
- Shah, K. R.; Sinha, Bikas K. (1996). "Row-column designs". In S. Ghosh and C. R. Rao. Design and analysis of experiments. Handbook of Statistics. 13. Amsterdam: North-Holland Publishing Co.. pp. 903–937. MR1492586. ISBN 0-444-82061-2.
- Raghavarao, Damaraju (1988). Constructions and Combinatorial Problems in Design of Experiments (corrected reprint of the 1971 Wiley ed.). New York: Dover. MR1102899.
- Street, Anne Penfold and Street, Deborah J. (1987). Combinatorics of Experimental Design. New York: Oxford University Press. pp. 400+xiv pp.. MR908490. ISBN 0198532563, 0-19-853255-5.
- J. H. van Lint, R. M. Wilson: A Course in Combinatorics. Cambridge University Press 1992,ISBN 0-521-42260-4, p. 157
External links
Design of experiments |
|
Scientific
Method |
Scientific experiment · Statistical design · Control · Internal & external validity · Experimental unit · Blinding
Optimal design: Bayesian · Random assignment · Randomization · Restricted randomization · Replication versus subsampling · Sample size
|
|
Treatment
& Blocking |
Treatment · Effect size · Contrast · Interaction · Confounding · Orthogonality · Blocking · Covariate · Nuisance variable
|
|
Models
& Inference |
Linear regression · Ordinary least squares · Bayesian
Random effect · Mixed model · Hierarchical model: Bayesian
Analysis of variance (Anova) · Cochran's theorem · Manova ( multivariate) · Ancova ( covariance)
Compare means · Multiple comparison ·
|
|
Designs:
Completely
Randomized |
Factorial · Fractional factorial · Plackett-Burman · Taguchi
Response-surface design · Polynomial & rational modeling · Box-Behnken · Central composite
Block · Generalized randomized block design (GRBD) · Latin square · Graeco-Latin square · Hyper-Graeco-Latin square · Latin hypercube
Repeated measures experiment · Crossover experiment ·
Randomized controlled trial · Sequential analysis · Sequential probability ratio test
|
|
Glossary · Category · Statistics portal · Statistical outline · Statistical topics |
|
Statistics |
|
Descriptive statistics |
|
Continuous data
|
Location
|
|
|
Dispersion
|
|
|
Shape
|
|
|
|
Count data
|
Index of dispersion
|
|
Summary tables
|
Grouped data · Frequency distribution · Contingency table
|
|
|
Pearson product-moment correlation · Rank correlation ( Spearman's rho, Kendall's tau) · Partial correlation · Scatter plot
|
|
Statistical graphics
|
Bar chart · Biplot · Box plot · Control chart · Correlogram · Forest plot · Histogram · Q-Q plot · Run chart · Scatter plot · Stemplot · Radar chart
|
|
|
|
Data collection |
|
Designing studies
|
Effect size · Standard error · Statistical power · Sample size determination
|
|
Survey methodology
|
|
|
|
Design of experiments · Randomized experiment · Random assignment · Replication · Blocking · Regression discontinuity · Optimal design
|
|
Uncontrolled studies
|
Natural experiment · Quasi-experiment · Observational study
|
|
|
|
Statistical inference |
|
|
Bayesian probability · Prior · Posterior · Credible interval · Bayes factor · Bayesian estimator · Maximum posterior estimator
|
|
Frequentist inference
|
|
|
Specific tests
|
Z-test (normal) · Student's t-test · F-test · Chi-square test · Pearson's chi-square · Wald test · Mann–Whitney U · Shapiro–Wilk · Signed-rank · Likelihood-ratio
|
|
|
Mean-unbiased · Median-unbiased · Maximum likelihood · Method of moments · Minimum distance · Maximum spacing · Density estimation
|
|
|
|
Correlation and regression analysis |
|
|
Pearson product-moment correlation · Partial correlation · Confounding variable · Coefficient of determination
|
|
|
Errors and residuals · Regression model validation · Mixed effects models · Simultaneous equations models
|
|
|
Simple linear regression · Ordinary least squares · General linear model · Bayesian regression
|
|
Non-standard predictors
|
Nonlinear regression · Nonparametric · Semiparametric · Isotonic · Robust
|
|
Generalized linear model
|
Exponential families · Logistic (Bernoulli) · Binomial · Poisson
|
|
Formal analyses
|
|
|
|
|
Data analyses and models for other specific data types |
|
|
Multivariate statistics
|
|
|
|
Decomposition · Trend estimation · Box–Jenkins · ARMA models · Spectral density estimation
|
|
Survival analysis
|
Survival function · Kaplan–Meier · Logrank test · Failure rate · Proportional hazards models · Accelerated failure time model
|
|
Categorical data
|
McNemar's test · Cohen's kappa
|
|
|
|
Applications |
|
Engineering statistics
|
Methods engineering · Probabilistic design · Process & Quality control · Reliability · System identification
|
|
Environmental statistics
|
|
|
Medical statistics
|
|
|
Social statistics
|
|
|
|
|
Category · Portal · Outline · Index |
|